Cox proportional hazards models
Cox proportional hazards models are used to model survival time using covariates. They are available in Excel using the XLSTAT statistical software.
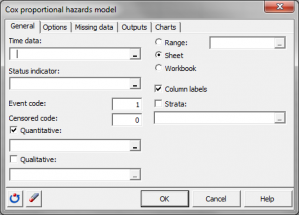
What are Cox proportional hazards models
The principle of the Cox proportional hazards model is to link the survival time of an individual to covariates. For example, in the medical domain, we are seeking to find out which covariate has the most important impact on the survival time of a patient.
Cox Models
A Cox model is a well-recognized statistical technique for exploring the relationship between the survival of a patient and several explanatory variables. A Cox model provides an estimate of the treatment effect on survival after adjustment for other explanatory variables. It allows us to estimate the hazard (or risk) of death, or other event of interest, for individuals, given their prognostic variables.
Interpreting a Cox model involves examining the coefficients for each explanatory variable. A positive regression coefficient for an explanatory variable means that the hazard for patient having a high positive value on that particular variable is high. Conversely, a negative regression coefficient implies a better prognosis for patients with higher values of that variable.
Cox’s method does not assume any particular distribution for the survival times, but it rather assumes that the effects of the different variables on survival are constant over time and are additive in a particular scale.
The hazard function is the probability that an individual will experience an event (for example, death) within a small time interval, given that the individual has survived up to the beginning of the interval. It can therefore be interpreted as the risk of dying at time t. The hazard function (denoted by λ(t,X)) can be estimated using the following equation:
λ(t,X) = λ0(t) exp(βX)
The first term depends only on time and the second one depends on X. We are only interested on the second term. If we only estimate the second term, a very important hypothesis has to be verified: the proportional hazards hypothesis. It means that the hazard ratio between two different observations does not depend on time. Cox developed a modification of the likelihood function called partial likelihood to estimate the coefficients β not taking into account the time dependent term of the hazard function:
log[L(β)] = Σi=1..n βXi - log[Σj=t(j)≥ t(i) exp(βXj)]
To estimate the β parameters of the model (the coefficients of the linear function), we try to maximize the partial likelihood function. Contrary to linear regression, an exact analytical solution does not exist. So an iterative algorithm has to be used. XLSTAT uses a Newton-Raphson algorithm. The user can change the maximum number of iterations and the convergence threshold if desired.
Strata in the Cox proportional hazards model
When the proportional hazards hypothesis does not hold, the model can be stratified. If the hypothesis holds on sub-samples, then the partial likelihood is estimated on each sub-sample and these partial likelihoods are summed in order to obtain the estimated partial likelihood. In XLSTAT, strata are defined using a qualitative variable.
Qualitative variables in the Cox proportional hazards model
Qualitative covariates are treated using a complete disjunctive table. In order to have independent variables in the model, the binary variable associated to the first modality of each qualitative variable has to be removed from the model. In XLSTAT, the first modality is always selected and, thus, its effect corresponds to a standard. The impacts of the other modalities are obtained relatively to the omitted modality.
Ties handling for Cox proportional hazards model
The proportional hazards model has been developed by Cox (1972) in order to treat continuous time survival data. However, frequently in practical applications, some observations occur at the same time. The classical partial likelihood cannot be applied. With XLSTAT, you can use two alternative approaches in order to handle ties:
- Breslow’s method (1974) (default method): The partial likelihood has the following form: log[L(β)] = Σi=1..T β Σl=1..diXl - di log[Σj=t(j)≥ t(i) exp(βXj)], where T is the number of times and di is the number of observations associated to time t(i).
- Efron’s method (1977): The partial likelihood has the following form: log[L(β)] = Σi=1..T β Σl=1..diXl - Σr=0..di-1 log [Σj=t(j)≥ t(i) exp(βXj) – r/di Σj=1..di exp(βXj)], where T is the number of times and i is the number of observations associated to time t(i).
If there are no ties, partial likelihoods are equivalent to Cox partial likelihood.
Variables selection for the Cox proportional hazard model
It is possible to improve the Cox proportional hazards model by selecting the variables being part of the model. XLSTAT offers two options to select the variables:
- Forward selection: The selection process starts by adding the variable with the largest contribution to the model. If a second variable is such that its entry probability is greater than the entry threshold value, then it is added to the model. This process is iterated until no new variable can be entered in the model.
- Backward selection: This method is similar to the previous one but starts from a complete model.
Results for the Cox proportional hazard in XLSTAT
Goodness of fit coefficients for the Cox proportional hazard model
The goodness of fit coefficients table displays a series of statistics for the independent model (corresponding to the case where there is no impact of covariates, beta=0) and for the adjusted model.
- Observations: The total number of observations taken into;
- DF: Degrees of freedom;
- -2 Log(Like.): The logarithm of the likelihood function associated with the model;
- AIC: Akaike’s Information Criterion;
- SBC: Schwarz’s Bayesian Criterion;
- Iterations: Number of iterations until convergence.
Statistical test of the Cox proportional hazard model
XLSTAT enables you to test the null hypothesis H0: beta=0:
The H0 hypothesis corresponds to the independent model (no impact of the covariates). We seek to check if the adjusted model is significantly more powerful than this model. Three tests are available: the likelihood ratio test (-2 Log(Like.)), the Score test and the Wald test. The three statistics follow a Chi2 distribution whose degrees of freedom are shown.
Model parameters
The parameter estimate, corresponding standard deviation, Wald's Chi², the corresponding p-value and the confidence interval are displayed for each variable of the model. The hazard ratios for each variable with confidence intervals are also displayed.
The residual table shows, for each observation, the time variable, the censoring variable and the value of the residuals (deviance, martingale, Schoenfeld and score).
Available charts for the Cox proportional hazard model
XLSTAT offers the following charts for the Cox proportional hazards model:
- Cumulative Survival distribution function (SDF),
- -Log(SDF),
- Log(-Log(SDF)),
- hazard function at mean of covariates,
- residuals.
analyze your data with xlstat
Included in
Related features