Generalized Procrustes Analysis (GPA)
Generalized Procrustes Analysis is used to obtain a consensual configuration among descriptions of products by several judges. Run GPA in Excel with the XLSTAT software.
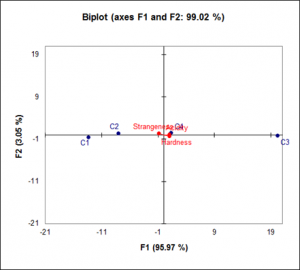
When to use Generalized Procrustes Analysis
Generalized Procrustean Analysis (GPA) is used in sensory data analysis prior to a Preference Mapping to reduce the scale effects and to obtain a consensual configuration. It also allows comparing the proximity between the terms that are used by different experts to describe products.
Principle of Generalized Procrustes Analysis
We define by configuration an n x p matrix that corresponds to the description of n objects (or individuals/cases/products) on p dimensions (or attributes/variables/criteria/descriptors).
We name consensus configuration the mean configuration computed from the m configurations. Procrustes Analysis is an iterative method that allows to reduce, by applying transformations to the configurations (rescaling, translations, rotations, reflections), the distance of the m configurations to the consensus configuration, the latter being updated after each transformation.
Let us take the example of 5 experts rating 4 cheeses according to 3 criteria. The ratings can go from 1 to 10. One can easily consider that an expert tends to be harder in his notation, leading to a shift to the bottom of the ratings, or that another expert tends to give ratings around the average, without daring to use extreme ratings. To work on an average configuration could lead to false interpretations. One can easily see that a translation of the ratings of the first expert is necessary, or that rescaling the ratings of the second expert would make his ratings possibly closer to those of the other experts.
Once the consensus configuration has been obtained, it is possible to run a PCA (Principal Components Analysis) on the consensus configuration in order to allow an optimal visualization in two or three dimensions.
There are two cases:
- If the number and the designation of the p dimensions are identical for the m configurations, one speaks in sensory analysis about conventional profiles.
- If the number p and the designation of the dimensions varies from one configuration to the other, one speaks in sensory analysis about free profiles, and the data can then only be represented by a series of m matrices of size n x p(k), k=1,2, …, m.
Algorithms for Generalized Procrustes Analysis used in XLSTAT
XLSTAT is the unique product offering the choice between the two main available algorithms: the one based on the works initiated by John Gower (1975), and the later one described in the thesis of Jacques Commandeur (1991). Which algorithm performs best (in terms of least squares) depends on the dataset, but the Commandeur algorithm is the only one that allows to take into account missing data; by missing data we mean here that for a given configuration and a given observation or row, the values were not recorded for all the dimensions of the configuration. The latter can happen in sensory data analysis if one of the judges has not evaluated a product.
Results for the Generalized Procrustes Analysis in XLSTAT
PANOVA table
Inspired from the format of the analysis of variance table of the linear model, this table allows you to evaluate the relative contribution of each transformation to the evolution of the variance. In this table are displayed the residual variance before and after the transformations, the contribution to the evolution of the variance of the rescaling, rotation and translation steps. The computing of the Fisher’s F statistic enables you to compare the relative contributions of the transformations. The corresponding probabilities help you to determine whether the contributions are significant or not.
Residuals
Residuals by object: This table and the corresponding bar chart allow to visualize the distribution of the residual variance by object. Thus, it is possible to identify for which objects the GPA has been the less efficient, in other words, which objects are the farther from the consensus configuration.
Residuals by configuration: This table and the corresponding bar chart allow you to visualize the distribution of the residual variance by configuration. Thus, it is possible to identify for which configurations the GPA has been the less efficient, in other words, which configurations are the farther from the consensus configuration.
Scaling factors for each configuration
Scaling factors for each configuration presented either in a table or a plot allow to compare the scaling factors applied to the configurations. It is used in sensory analysis to understand how the experts use the rating scales.
Results of the consensus test
The number of permutations that have been performed, the value of Rc which corresponds to the proportion of the original variance explained by the consensus configuration, and the quantile corresponding to Rc, calculated using the distribution of Rc obtained from the permutations are displayed to evaluate the effectiveness of the Generalized Procrustean Analysis. You need to set a confidence interval (typically 95%), and if the quantile is beyond the confidence interval, one concludes that the Generalized Procrustean Analysis significantly reduced the variance.
Results of the dimensions test
For each factor retained at the end of the PCA step, the number of permutations that have been performed, the F calculated after the Generalized Procrustean Analysis (F is here the ratio of the variance between the objects, on the variance between the configurations), and the quantile corresponding to F calculated using the distribution of F obtained from the permutations are displayed to evaluate if a dimension contributes significantly to the quality of the Generalized Procrustean Analysis. You need to set a confidence interval (typically 95%), and if the quantile is beyond the confidence interval, one concludes that factor contributes significantly. As an indication are also displayed, the critical values and the p-value that corresponds to the Fisher’s F distribution for the selected alpha significance level. It may be that the conclusions resulting from the Fisher’s F distribution is very different from what the permutations test indicates: using Fisher’s F distribution requires to assume the normality of the data, which is not necessarily the case.
Results for the consensus configuration
- Objects coordinates before the PCA: This table corresponds to the mean over the configurations of the objects coordinates, after the Generalized Procrustean Analysis transformations and before the PCA.
- Eigenvalues: If a PCA has been requested, the table of the eigenvalues and the corresponding scree-plot are displayed. The percentage of the total variability corresponding to each axis is computed from the eigenvalues.
- Correlations of the variables with the factors: These results correspond to the correlations between the variables of the consensus configuration before and after the transformations (Generalized Procrustean Analysis and PCA if the latter has been requested). These results are not displayed on the circle of correlations as they are not always interpretable.
- Objects coordinates: This table corresponds to the mean over the configurations of the objects coordinates, after the transformations (Generalized Procrustean Analysis and PCA if the latter has been requested). These results are displayed on the objects charts.
Results for the configurations after transformations
- Variance by configuration and by dimension: This table allows to visualize how the percentage of total variability corresponding to each axis is divided up for the configurations.
- Correlations of the variables with the factors: These results, displayed for all the configurations, correspond to the correlations between the variables of the configurations before and after the transformations (GPA and PCA if the latter has been requested). These results are displayed on the circle of correlations.
- Objects coordinates (presentation by configuration): This series of tables corresponds to the objects coordinates for each configuration after the transformations (GPA and PCA if the latter has been requested). These results are displayed on the first series of objects charts.
- Objects coordinates (presentation by object): This series of tables corresponds to the objects coordinates for each configuration after the transformations (GPA and PCA if the latter has been requested). These results are displayed on the second series of objects charts.
analyze your data with xlstat
Related features