STATIS
Use STATIS to analyze multiple configurations of objects / quantitative variables to study and visualize the links between the objects as well as the agreements between the configurations.
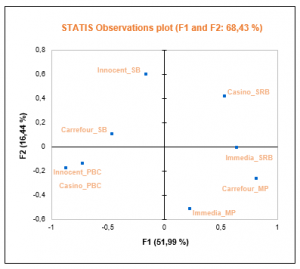
What is the STATIS method?
The STATIS method is a multi-configuration data analysis method most used in sensometry. The configurations can be various assessors, subjects or judges. STATIS can be particularly used in the case of projective mapping / Napping, conventional profiling, and free choice profiling. The great interest of STATIS is that atypical configurations have a smaller weight than this of central configurations. Therefore, the analysis best reflects the general point of view and not those atypical configurations.
There are several applications of STATIS, including:
- Study and visualization of objects in the main plans;
- Study the links between the configurations, especially to find the most atypical.
Principle of STATIS
STATIS is a method working on the scalar matrix of each configuration, thus making it possible to work with configurations with different numbers of columns. Its aim is to form a consensus configuration that reflects at best the different configurations. This consensus can then be projected on different axes. If the information associated with 2 or 3 first axes represents a sufficient percentage of the total variability of the consensus, the objects will be able to be represented on a 2- 3-dimensional chart, thus making interpretation much easier.
Need to choose the appropriate sensory data analysis tool for your study? Check our guide.
What are the options for the STATIS analysis in XLSTAT?
Structure of the data
Two cases exist:
1. The number of the p variables is identical for the m configurations.
2. The number p of the variables varies from one configuration to the other.
For data entry, XLSTAT asks you to select a configuration corresponding to the m contiguous configurations and to give the case of structure.
Scaling and global scaling
If the data within a configuration are not on the same scale, it is advisable to scale (reduce) the variables of each configuration. For example, this is not the case for ratings between 0 and 20, but it is advised if some notes lay between 0 and 10 and others between 0 and 20.
Classically, the overall reduction of each configuration is recommended. It allows to put all the configurations on an equal footing in term of variance. For example, in the case, of configurations where the attributes are noted between 0 and 20 by assessors, it will remove scale factors between the assessor that only notes between 5 and 15 and the assessor that uses the full scale of notes.
Interpreting the results of the STATIS analysis in XLSTAT
The representation of objects in the k factors space allows us to visually interpret the proximities between objects, with precautions.
We can consider that the projection of an object on a plan is reliable if the object is far from the center of the graph.
Number of factors
Two methods are commonly used to determine how many factors must be retained for the interpretation of the results:
- Watch the decreasing curve of eigenvalues. The number of factors to be kept corresponds to the first turning point found on the curve.
- We can also use the cumulative variability percentage represented by the factor axes and decide to use only a certain percentage.
Graphical representations
However, these representations are only reliable if the sum of the percentages of variability associated with the axes of the representation space is sufficiently high.
If this percentage is high (80%), the representation can be considered reliable. If the percentage is low, it is recommended to produce representations on several axis pairs to validate the interpretation made on the two first factor axes.
Which are the results of the STATIS analysis in XLSTAT?
Eigenvalues: The eigenvalues and corresponding chart (scree plot ) are displayed.
Consensus coordinates: Consensus coordinates in the factors space are displayed, with the corresponding charts (depending on the number of factors chosen).
RV matrix: The matrix of RV coefficients between all configurations is displayed. The coefficient RV is a coefficient of similarity between two configurations included between 0 and 1. The closer it is to 1, the stronger the similarity. This matrix is used by STATIS to calculate the weights of the configurations.
Scaling factors: The scaling factors are displayed with the associated bar chart. The larger a scale factor in a configuration, the smaller the scale of the configuration used. This table is used in sensory analysis to understand how assessors use differently the rating scales.
Weights: The weights calculated by STATIS are displayed, with the associated bar chart. The greater the weight, the more the configuration contributed to the consensus. Knowing that STATIS gives more weight to the closest configurations from a global point of view, a much lower weight than the others will mean that the configuration is atypical.
Consensus configuration: The consensus configuration is displayed. It corresponds to the weighted average of the scalar product matrices of the initial configurations (possibly reduced by variable and/or globally).
Homogeneity: The homogeneity of the configurations is displayed. It is a value between 1/m (m being the number of configurations) and 1, which increases with the homogeneity of the configurations.
RV config/consensus: The RV coefficients between the configurations and the consensus are displayed, with the associated bar chart. Like the weights of STATIS, these coefficients make it possible to detect atypical configurations. The advantage of these coefficients is that they are between 0 and 1, so they are easier to interpret than the weights.
Global error: The global error of the STATIS criterion is displayed. It corresponds to the sum of all residuals (which can be presented by configuration or object).
Residuals by configuration: This table and the corresponding bar chart make it possible to visualize the distribution of the residuals by configuration. It is thus possible to identify for which configurations STATIS has been less efficient, or in other words, which configurations stand out the most from the consensus.
Residuals by object: This table and the corresponding bar chart make it possible to visualize the distribution of the residuals by object. It is thus possible to identify for which objects STATIS has been less efficient, or in other words, which objects stand out the most from the consensus.
Correlations: The correlations among the factors and the initial variables, as well as the correlation circle are displayed. This chart shows the links between the different variables and the factors.
Objects coordinates (presentation by configuration): This series of tables corresponds to the coordinates of the objects for each configuration, after the optional scaling and global scaling then the projection on the factors. The presentation is made by configuration.
Objects coordinates (presentation by object): This series of tables corresponds to the coordinates of the objects for each configuration, after the optional scaling and global scaling then the projection on the factors. The presentation is made by object.
analyze your data with xlstat
Related features