Statistical Power for Cox model
Ensure optimal power or sample size using power analysis. Power for Cox regression is available in Excel using the XLSTAT statistical software.
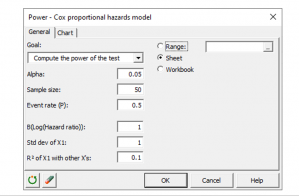
Statistical Power for Cox model
XLSTAT-Life Sciences offers a tool to apply the proportional hazards ratio Cox regression model. XLSTAT-Power estimates the power or calculates the necessary number of observations associated with this survival analysis model.
What is Statistical Power for Cox model?
What is Statistical Power?
When testing a hypothesis on data using a statistical test, there are several decisions to take:
- The null hypothesis H0 and the alternative hypothesis Ha.
- The statistical test to use.
- The type I error also known as alpha. It occurs when one rejects the null hypothesis when it is true. It is set a priori for each test and is 5%.
The type II error or beta is less studied but is of great importance. In fact, it represents the probability that one does not reject the null hypothesis when it is false. We cannot fix it up front, but based on other parameters of the model we can try to minimize it. The power of a test is calculated as 1-beta and represents the probability that we reject the null hypothesis when it is false.
We therefore wish to maximize the power of the test. The XLSTAT-Power module calculates the power (and beta) when other parameters are known. For a given power, it also allows to calculate the sample size that is necessary to reach that power because they are dependent on each other.
The statistical power calculations are usually done before the experiment is conducted. The main application of power calculations is to estimate the number of observations necessary to properly conduct an experiment. This will enable us to choose the size of our data set on which we will conduct the survival study.
What is the Cox model?
The Cox survival model is based on the hazard function which is the probability that an individual will experience an event (for example, death) within a small time interval, given that the individual has survived up to the beginning of the interval. It can therefore be interpreted as the risk of dying at time t. The hazard function (denoted by λ(t,X)) can be estimated using the following regression equation: λ(t,X) = λ0 (t) exp(β1X1 + … + (βpXp) The first term depends only on time and the second one depends on X. We are only interested in the second term. If all coefficients βi are equal to zero then there is no hazard factor.
The goal of the Cox model is to focus on the relations between the coefficients βi and the hazard function. It is called the proportional hazards model because it is based on the assumption that the coefficients make the hazards increase propotionally by exp(βi ), for each Xi variable every time it changes modalities (increase in age category...).
The test used in XLSTAT-Power is based on the null hypothesis that the β1 coefficient is equal to 0. That means that the X1 covariate is not a hazard factor.
The hypothesis to be tested is:
- H0: β1 = 0
- Ha: βi ≠ 0
Power is computed using an approximation which takes values depending on the normal distribution. Other parameters used in this approximation are: the event rate, which is the proportion of uncensored individuals, the standard deviation of X1, the expected value of β1 known as B(log(hazard ratio)) and the R² obtained with the regression between X1 and the other covariates included in the Cox model.
Options for Statistical Power for Cox model using the XLSTAT software
You can choose to calculate the size of your data sample based on a set power, or to calculate the power reachable when using a set sample size. Anyway, a simulation plot of the power depending on the sample size is displayed.
You can also select your type I statistical error alpha as well as the event rate (unit rate without the censored units) and the B(log(hazard ratio)) coefficient.
Finally, you can enter the R² obtained with a regression between X1 and the other covariates (explanatory variables) of the model.
Calculating B for the Cox model
The B(log(hazard ratio)) is an estimation of the coefficient β1 of the following equation: log(λ(t|X) / λ0(t)) = β1X1 + … + βkXk . β1 is the change in logarithm of the hazard ratio when the X1 variable is incremented by one unit (all other explanatory variables remaining constant). We can use the hazard ratio instead of the log. For a hazard ratio of 2, we will have B = log(2) = 0.693.
Obtaining the R² between X1 and the other explanatory variables of the model
In order to obtain the R² between the X1 variable and the others, you can run a Linear regression on your variables. The R² statistical indicator is always displayed in the results after the fit of the models.
Results for Statistical Power for Cox model in XLSTAT
The displayed results include your inputs (parameter values to compute power and required data sample size), as well as the parameters obtained by the calculation. If you choose to display it while setting up your analysis, you can also see the simulation plot which links the set sample size to the power.
Calculating sample size for the Cox model taking statistical power into account
To calculate the number of observations required, XLSTAT uses an algorithm that searches for the root of a function. It is called the Van Wijngaarden-Dekker-Brent algorithm (Brent, 1973). This algorithm is adapted to the case where the derivatives of the function are not known. It tries to find the root of:
power (N) - expected_power
We then obtain the size N such that the test has a power as close as possible to the desired power.
You can try this feature yourself by following this Statistical Power for Cox model tutorial.
analyze your data with xlstat
Related features